The 411 on Data Cleansing, Modeling, and Governance for Marketers
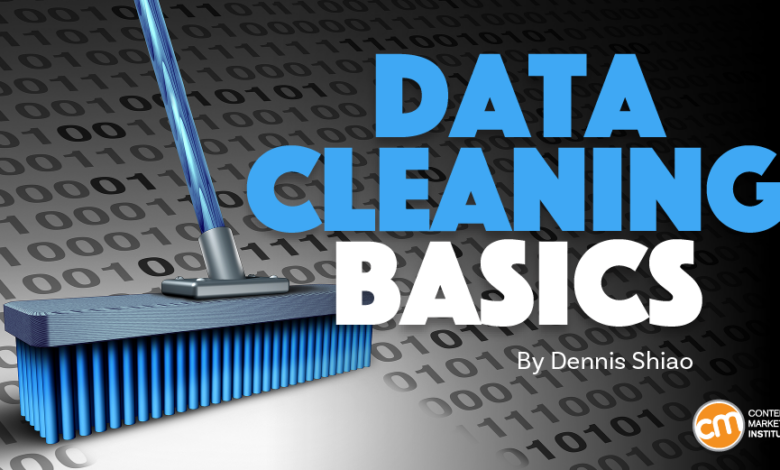
You can access a wealth of marketing-related data, from web analytics and customer journey behavior to competitor analysis and product usage.
However, if the data isn’t clean, you can’t really exploit its value. Or worse yet, you could steer your marketing in the wrong direction and see diminishing returns.
James Huntsenior consultant at Vivanti, says data cleaning and modeling is essential to extracting value and gaining knowledge and wisdom from information. In his presentation to Marketing Analytics and Data Science Conference, it details why it’s necessary, the basics of data cleansing, and the role of governance and observability.
What is data modeling?
Data models transform data into something useful, and you need to understand data modeling so you can understand the best cleaning options. James explains that data modeling involves three parts: additive, contextual and domain.
Additive means you let machines figure out how to standardize data. You don’t manually “correct” the data, for example by lowercaseing sporadic names to uppercase on a spreadsheet. This would effectively amount to data destruction because, as James says: “As humans, we’re really bad at doing the same thing twice. »
Context organizes data to tell a story. You are not adding new information; you impute existing data. For example, the context of a sales transaction might include marketing emails the buyer saw, social media content the buyer interacted with, and other products the buyer viewed.
Domain is the set of all possible data values for a given element. This can be qualitative and quantitative. James highlights these five common domain types:
Identify — a unique value that distinctly and discreetly identifies someone, such as an email address, social security number, or customer ID
Nominative — an additional identity not strong enough to stand alone, such as a person’s full name or the name of a product
Categorical — grouping across arbitrary boundaries, such as customer type or industry; often used for cohort subdivision
Monetary — the currency that can be compared, ordered, aggregated and disaggregated, such as order total or unit price
Temporal — a point or period of dates and times, such as registration date, last purchase date, or loyalty period
With this fundamental understanding of modeling, you are ready to learn more about data cleaning.
What types of data cleaning exist?
James details the three types of data cleansing: mechanical and explicit mappings, as well as models and rules:
With mechanical cleaningthe data is cleaned without changing the meaning of the information, for example by normalizing the case of names and removing unnecessary spaces. “These are all things that I can do on my own as a data engineer and that no one gets upset about,” James says. “No one says, ‘Well, you took the spaces out of his first name, so he’s a different person.’
Explicit mapping uses an activity called “cardinality reduction” to decrease the number of unique values associated with an attribute. It simplifies the dataset by grouping values while retaining relevant information. These datasets are easier to manage and can improve model performance.
For example, James says, maybe a customer status field starts with two values: active and inactive. Over time, the scope has expanded to include suspended, pending, and prospective options. Explicit cleanup of the mapping can move the client status from “suspended” to “active”.
A cleaning for models and rules identifies and corrects inconsistencies, inaccuracies, or errors in data based on identifiable structures (i.e., patterns) and constraints (i.e., rules).
Standard templates include data such as email addresses, date strings, and phone numbers. Deviations from this structure indicate data that needs cleaning.
Rules refer to logical conditions or constraints. So, for example, if the monetary data of an insurance policy exceeds its maximum value, the entry must be cleaned.
James says you can also set rules and templates to map the customer journey. Let’s say a brand doesn’t care how many times someone opens and clicks on their email. Instead, it is concerned with identifying who is likely to purchase within an email marketing campaign. It could establish rules to clean the data for this purpose.
For example, all sent emails would be labeled “E” and all clicks would be labeled “C”, while an order would be recognized as “O”. These rules group the data, which is therefore very useful for the brand and its marketing objectives.
What is the role of governance in data cleansing?
“Every time you clean data, you make a decision. You decide what is relevant; you decide what is important. You decide what to keep and what to surface,” says James.
You should document these data cleansing decisions in an internal repository, such as a spreadsheet, or use a version control system like open source Git.
Every decision must answer these four questions:
What decision was made?
When was it made? This specific reference facilitates historical analysis.
Who made the decision?
Why was this decision made? It is useful to inform future actions. For example, if the decision was made because of a government update, it probably isn’t possible to reverse it. But if the decision was made because the data team thought it was a better way to go, reversing course may still be a viable option, James says.
Let’s go back to the example of collapsing customer status fields so that “suspended” status is grouped into “active” customers. Here is how this decision could be recorded:
“Customers with a “suspended status” are still considered active as of October 22, 2024. The decision was made by James Hunt as a mapping analysis showed that customer behaviors can be better assessed by their active or inactive status .
Humans are essential to the governance process, James says. Computer-generated algorithms can suggest data cleaning steps, but a human must be in the know to review the suggestions and approve or reject them.
What is observability?
Even after you set rules and patterns to ensure data cleanliness, some data will not meet these parameters. Instead of passing on this data or automatically cleaning it, you should embrace observability, which James says is 10 times more important than governance.
Bringing up the metadata from your data cleansing might look like this example from a client of James. Data cleansing rules set a lower limit on the size of policies to detect bad data. This worked well for about six months until a policy entered the system with a lower limit than set in the rules.
James flagged this recording, then asked the customer, “Do you want us to adjust the limit?” » The customer said yes and the lower limit data rule was updated.
“We figured this out through the observability loop by saying, ‘This is what we expect the data to look like.’ It didn’t look like that when we cleaned it. We were not comfortable making this decision (without customer input). And that’s what observability is going to give you,” says James.
Having the right observability practices can save you hours, days, weeks, months, and more embarrassment, he notes.
Are you ready to continue cleaning the data?
Now that you’ve learned about data modeling, cleaning, governance, and observability, you’re ready to apply it to your marketing if you have:
Datasets where data integrity is not intact or perfect Datasets with a high number of unique values (i.e. where reducing cardinality can make processing and analysis easier )
Where would you find this data? This can come from a multitude of sources, such as:
CRM Platforms Customer Contact Records Customer Questionnaires and Feedback Forms Survey Responses Web Analytics Customer Behaviors Product or Platform Insights Competitor Analytics
Start with those that would benefit most from one or more of the three types of data cleansing, appropriate governance, and observability. Then you can decide whether or not to collaborate with your organization’s data teams to help you.
MADS 2024 is over, but you can still enjoy all the learning and inspiration. A digital pass gives you access to recordings of Seth Stephens-Davidowitz’s keynote and in-depth sessions from Etsy’s Vishwa Bhuta, Google’s Suraj Rajdev, ReflexAI’s John Callery-Coyne, and many other experts. Sign up for a MADS Digital Pass today to make every minute of access count — access expires January 31, 2025. (Remember to use code DAA200 to save $200).
HANDPICKED RELATED CONTENT:
Cover image by Joseph Kalinowski/Content Marketing Institute