How to Overcome 5 Barriers to Adopting AI in Marketing Analytics
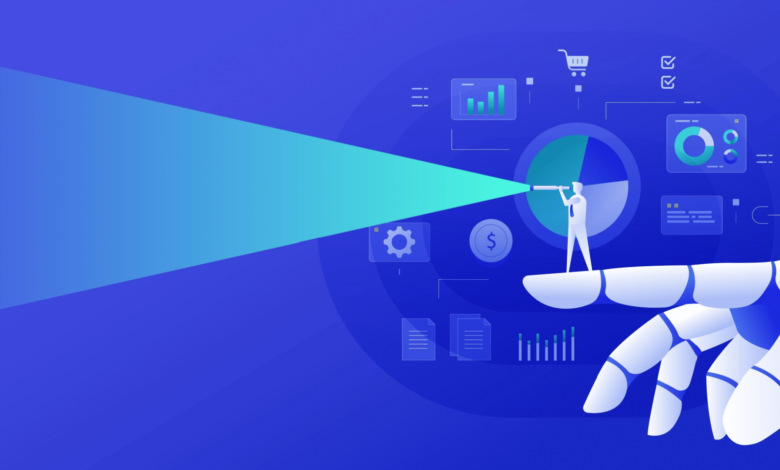
AI is poised to transform the way we work, but its full potential remains untapped. In the field of marketing analytics, AI promises to revolutionize the field by:
Enable significant performance improvements. Unleash untold operational efficiencies. Enhance layers of intelligence and interpretation to improve actionable insights and analytics.
Given the potential for transformational gains, widespread adoption of AI should be the norm in marketing analytics. Why not ? What obstacles prevent this change? More importantly, what can organizations and their teams do to change this? Here we provide practical answers to these questions.
Why AI Adoption in Marketing Analytics Is Lagging
Let’s start with the blockers, as highlighted by IBM’s AI Adoption Index 2023. They identify five key obstacles:
Difficulty integrating and scaling. Complexity of the underlying data. Costs. Limited skills. Ethical concerns.
These challenges are important, but we view them more as obstacles than insurmountable barriers: obstacles that can be overcome with a use case-based approach to AI deployment.
Over the past year, we’ve applied this approach to nearly a dozen brands, achieving rapid ROI and substantial performance improvements. Here’s how.
Dig Deeper: The AI-powered path to smarter marketing
Define your use case
Sometimes use cases are self-evident. For example, a large retailer we work with is facing a customer churn problem, for which an AI-based approach to predicting churn could provide significant business value.
Other times, the most relevant use case isn’t as obvious. In these cases, creating a use case catalog helps prioritize opportunities. This catalog lists potential AI-enhanced use cases and scores them based on impact, scale, and effort required.
Here are some fundamental use cases of AI in marketing analytics that we have encountered:
Data mapping and transformation to accelerate data integration. Metadata generation and data classification to enrich datasets. Predictive scoring and segmentation to drive customer action. AI-powered cluster analytics for rapid audience discovery. Message and channel optimizations to increase response rates. AI assistants enabling natural language data queries.
These examples illustrate how AI can drive substantial business value. Once use cases are defined, the focus should be on removing barriers to implementation.
Dig Deeper: AI and Machine Learning in Marketing Analytics: A Revenue-Driven Approach
Overcoming obstacles: practical solutions
1. AI integration and scaling
The first hurdle can be overcome by focusing on a high-value, low-effort use case, as highlighted by the use case catalog approach. For example, our churn prevention strategy for one client involved using AI-based customer intelligence to trigger emails for high-risk customers. This solution was seamlessly integrated into existing workflows, demonstrating how targeted use cases simplify scaling efforts.
2. Manage data complexity
The complexity of the underlying data is the most common obstacle we encounter. The aphorism “Don’t let the good be the enemy of the great” is quite apt. Data is never perfect. The best approach is to put aside the quest for perfection and focus on the data that matters.
Website interaction data and customer transaction data are two types of data commonly available in most businesses. They are particularly powerful for creating AI-based segmentation models for propensity, engagement, loyalty, and churn. Additionally, AI-enabled data preparation and cleaning can automate tedious tasks, enabling faster and more comprehensive data access.
Dig Deeper: 4 Ways to Fix Bad Data and Improve Your AI
3. Justify the expense
Spending problems often stem from a fundamental misunderstanding of value creation. Implementing AI in marketing analytics requires investment. This can range from a modest $50,000 to start, to seven-figure sums for more ambitious projects. However, these expenses constitute an investment and not just an expense.
Return on investment can be predicted, quantified and measured. By focusing on specific use cases, it is easier to build a solid business case for ROI to justify the investment. For example, AI-based segmentation and scoring typically yields 10-15% improvements. A brand that invests $20 million in outbound marketing could see an annual return of $2-3 million, making a compelling argument for investing in AI.
4. Address skills gaps
Expanding the pool of available expertise can help meet limited skills. Although few professionals have both the technical skills and knowledge to deploy AI for marketing analytics, this problem is primarily internal to the company. The solution is to outsource the expertise.
In a rapidly changing environment, where specialized skills are both scarce and necessary, it is often impractical for companies to develop these capabilities in-house. Partnering with a specialist to create tailor-made AI marketing analytics applications is the most effective and least risky approach. These efforts can eventually become owned assets, but without the immediate burden of building and implementing them internally.
5. Manage ethical and legal concerns
The final obstacle, ethical concerns, stands out from the previous four. Although ethical considerations in AI are serious and impactful, we have not seen them pose a major barrier to the adoption of AI in marketing analytics. The most common blocker is practical: legal and compliance issues.
Legal and compliance teams are particularly concerned about generative AI, where fears of inappropriate or off-brand content, as well as copyright and intellectual property risks, can significantly slow down or even stop advertising initiatives. ‘AI.
Overcoming AI adoption challenges with use cases
Ultimately, each organization must establish its own governance and controls for AI adoption. For starters, focusing on high-impact, low-risk use cases has proven effective. For example:
Using generative AI to standardize and categorize campaign names across all marketing channels provides high utility and time savings with minimal risk. Likewise, using machine learning to predict future client actions and outcomes is a value-driven use case that most legal teams – industry regulations aside – would not object to not.
Paving the way for AI transformation in marketing analytics
AI is transformational and will revolutionize marketing analytics. A use case-based approach provides a clear roadmap for overcoming barriers to adopting AI in marketing analytics. This measured strategy paves the way for sustainable AI integration, builds trust among internal teams, and fosters AI expertise within the organization.
Marketing analytics leaders who adopt these strategies will be well-positioned to improve performance, streamline operations, and cultivate a responsive, data-driven culture ready to harness the potential of AI.
Dig Deeper: Why Causal AI is the Answer to Smarter Marketing
Contributing authors are invited to create content for MarTech and are chosen for their expertise and contribution to the martech community. Our contributors work under the supervision of the writing and contributions are checked for quality and relevance to our readers. The opinions they express are their own.